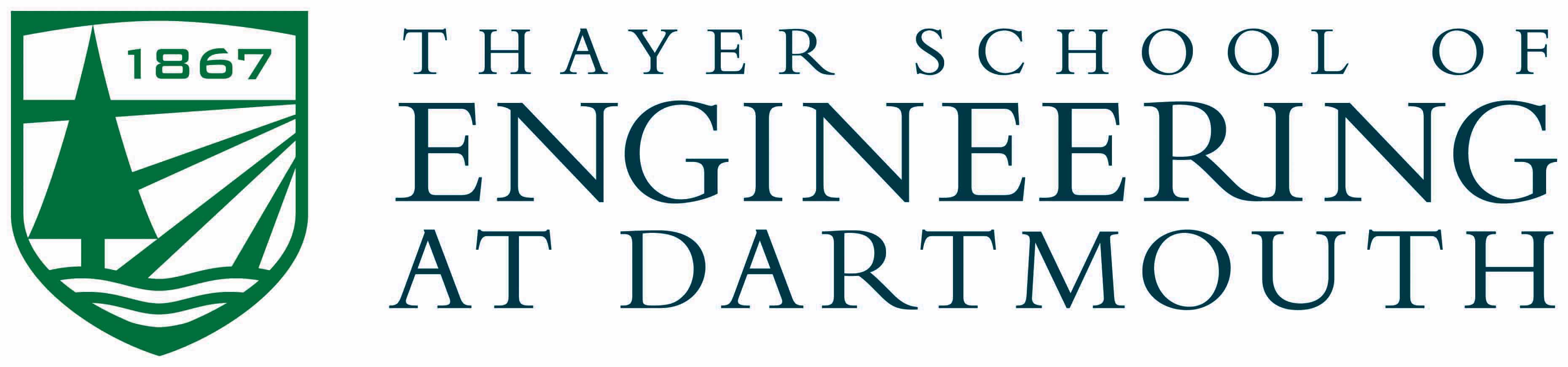
ENGS 88 Honors Thesis (AB Students)
Degree Program
A.B.
Year of Graduation
2020
Faculty Advisor
Geoffrey Luke
Document Type
Thesis (Senior Honors)
Publication Date
Spring 6-8-2020
Abstract
Compressed ultrafast photography (CUP) is a cutting-edge imaging technique that uses a variation of the traditional streak camera to obtain video at 100 billion frames per second with a single exposure. In order to achieve this level of temporal detail, CUP leverages compressed sensing (CS). Compressed sensing theory states that a compressed representation of an image can be directly acquired using a non-adaptive measurement matrix so long as the encoding matrix follows certain properties such as restrictive isometry and incoherence. This compressed representation of the original scene can later be reconstructed back into the original form. CUP applies CS by direly collecting an encoded image to represent an entire video. The image can then be reconstructed into the original frames using a time-consuming iterative reconstruction algorithm known as two-step iterative shrinking and thresholding. Research has shown that deep learning offers many promising advantages over standard iterative CS reconstruction algorithms both in speed and quality. While there are several applications of deep learning for CS reconstruction, all of these were created to reconstruct a single image and would not be directly applicable to CUP imaging. CUP-NET, a convolutional neural network based on the U-Net architecture, was trained, validated and tested using simulated CUP data. CUP-NET reconstructed videos 40,000x faster and with 2.5-3x better quality compared to TwIST. These results suggest that what was once a time-consuming and inexact imaging technique could become a real-time and extremely accurate framework for ultrafast imaging.
Dartmouth Digital Commons Citation
Parker, Matthew, "CUP-Net: Compressed Ultrafast Photography Using Convolutional Neural Networks" (2020). ENGS 88 Honors Thesis (AB Students). 15.
https://digitalcommons.dartmouth.edu/engs88/15
Included in
Artificial Intelligence and Robotics Commons, Computer Engineering Commons, Signal Processing Commons