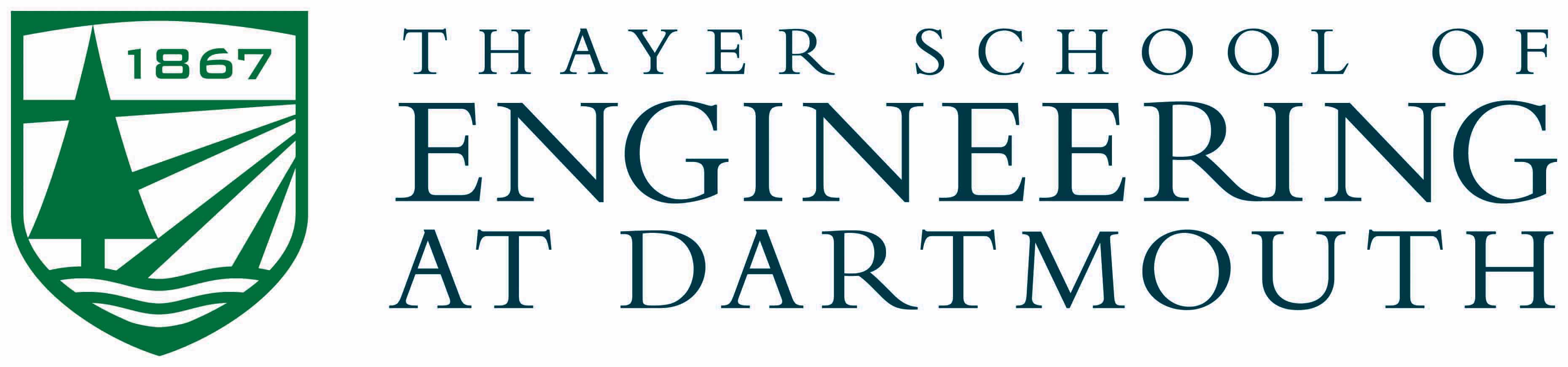
ENGS 89/90 Reports
Degree Program
B.E.
Year of Graduation
2022
Sponsor
Dr. Erik Kobylarz, Lebanon, NH
Project Advisor
Kofi Odame
Instructor
Laura Ray
Document Type
Report
Publication Date
2022
Abstract
Treatment plans for epilepsy depend on seizure frequency, duration, and type. Seizure monitoring in non-clinical settings relies on unreliable self-reports. Our sponsors have developed a suite of non-cerebral sensors which detects seizures with high specificity, though personalization of the suite may optimize results. Eye and head motion, not currently measured in the sensor suite, are indicative of certain seizure types, though state-of-the-art eye tracking devices have high power consumption and may not be suitable for extended monitoring. To inform future development of a low-power prototype for potential addition to the sensor suite, we gathered data from 8 subjects using a commercial eye-tracker (Pupil Core) and Inertial Measurement Unit (IMU), for non-seizure behaviors (technology use, eating, and conversation) and simulated seizure activity. Data processing using linear discriminant analysis (LDA) showed high separability between seizure and non-seizure data with features derived from head accelerometry and low-resolution eye position classification (area under the receiver operating characteristic curve was .98 and F1 score was .86 for eye position mapped to a 3x3 grid). The experimental protocol and data processing scripts we have provided allow for easy replication of our methodology to evaluate future hardware against these benchmark results. Additionally, adding artificial noise to Pupil Core data revealed that tracking the pupil within 2.75 mm was tolerable before separability scores significantly decreased. These results are promising for the use of eye and head motion data to identify certain seizure presentations, without requiring the high precision of current technology.
Level of Access
Restricted: Campus/Dartmouth Community Only Access
Dartmouth Digital Commons Citation
Provost, Brian and Vonderheide, David, "Eye Tracking for Seizure Detection" (2022). ENGS 89/90 Reports. 57.
https://digitalcommons.dartmouth.edu/engs89_90/57
Restricted
Available to Dartmouth community via local IP address.