Kernel-Based Regularized Least Squares in R (KRLS) and Stata (krls)
Document Type
Article
Publication Date
7-1-2017
Publication Title
Journal of Statistical Software
Department
Department of Government
Additional Department
Quantitative Social Science Program
Abstract
The Stata package krls as well as the R package KRLS implement kernel-based regularized least squares (KRLS), a machine learning method described in Hainmueller and Hazlett (2014) that allows users to tackle regression and classification problems without strong functional form assumptions or a specification search. The flexible KRLS estimator learns the functional form from the data, thereby protecting inferences against misspecification bias. Yet it nevertheless allows for interpretability and inference in ways similar to ordinary regression models. In particular, KRLS provides closed-form estimates for the predicted values, variances, and the pointwise partial derivatives that characterize the marginal effects of each independent variable at each data point in the covariate space. The method is thus a convenient and powerful alternative to ordinary least squares and other generalized linear models for regression-based analyses.
DOI
10.18637/jss.v079.i03
Dartmouth Digital Commons Citation
Jeremy, Ferwerda; Hainmueller, Jens; and Hazlett,, Chad J., "Kernel-Based Regularized Least Squares in R (KRLS) and Stata (krls)" (2017). Dartmouth Scholarship. 164.
https://digitalcommons.dartmouth.edu/facoa/164
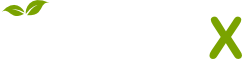
- Citations
- Policy Citations: 1
- Citation Indexes: 65
- Usage
- Abstract Views: 34
- Captures
- Readers: 86