Student Co-presenter Names
Daniel Carstensen, Kunal Jha, Ansh Patel
Files
Download Full Text (3.7 MB)
Student Class
2024
Student Affiliation
Junior Research Scholar
Author ORCID Identifier
https://orcid.org/0000-0001-7613-4732
First Advisor
Jeremy Manning
First Advisor Department
Department of Psychological and Brain Sciences
Description
Human cognition is fundamentally a network phenomenon: our thoughts, sense of self, and our other brain functions reflect coordinated interactions between our brain’s constituent cells and systems. These interactions change over time alongside our experiences, goals, and internally driven thoughts. Whereas early work on neural decoding attempted to infer internal representations by considering univariate (single region) or multivariate (patterns of regions) activity patterns, later studies have treated patterns of network interactions as a core unit of neural representation and computation. The field has also started to consider the potential role of higher order network interactions. If two regions, A and B, adjust their interactions over time in response to ongoing task demands, and two other regions, C and D, also adjust their interactions over time, second order interactions denote potential associations between the A-B interactions and C-D interactions. In the general case, order n interactions reflect associations between networks of order n - 1 interactions. In a recent study, our team found that meaningful high-order interactions between brain networks spontaneously emerge during deep cognitive processing, and they spontaneously dissociate during less demanding or focused cognition. Here we wondered if other sorts of networks might show a similar pattern. We applied a model of high-order network dynamics to a financial dataset comprising historical stock quotes from the S&P 500. We estimated which orders of interactions were most reflective of the “true” system dynamics by using different sets of features (made of network patterns at different orders) to predict future states of the market. We compared how informative different orders of interactions in financial data were at predicting future states of the S&P 500 to how informative different orders of brain interactions were in different experimental conditions in a neuroscientific dataset. We found that, like biological brains, financial markets appear to contain meaningful high-order interactions that change over time with market conditions, important historical events, and so on.
Publication Date
5-24-2023
Keywords
computational neuroscience, network models
Disciplines
Quantitative Psychology | Statistical Models
Dartmouth Digital Commons Citation
Jha, Kunal; Carstensen, Daniel; Patel, Ansh; and Manning, Jeremy R., "Exploring high-order network dynamics in brains and stock markets" (2023). Wetterhahn Science Symposium Posters 2023. 4.
https://digitalcommons.dartmouth.edu/wetterhahn_2023/4
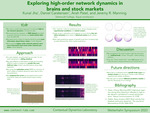
Restricted
Available to Dartmouth community via local IP address.