Files
Download Full Text (440 KB)
Student Class
2027
Student Affiliation
WISP Intern
First Advisor
Alireza Soltani
First Advisor Department
Department of Psychological and Brain Sciences
Description
Reinforcement learning is the process through which one learns the values of their actions and make adaptive decisions to maximize their reward. In naturalistic environments, this process can be challenging, as (1) choices can have long-term consequences and (2) choice options can have many features.
Previous studies showed that humans used successor representations to learn cognitive maps for planning. Other studies demonstrated that humans used feature-based learning and selective attention to learn the values of multi-featured stimuli. However, the interaction between these processes is largely unexplored.
To investigate this interaction, we designed a novel multidimensional two-step task, as well as six computational models representing different learning strategies. Using these models, we made concrete predictions about human behavior in our task paradigm, which can be tested in future experiments.
Publication Date
Spring 5-22-2024
Keywords
Reinforcement learning, computational modeling, decision-making, successor representation, feature-based learning, selective attention
Disciplines
Computational Neuroscience
Dartmouth Digital Commons Citation
Choi, Seoyoon; Wang, Chong; and Soltani, Alireza, "Computational modeling of human sequential decision making in high-dimensional environments" (2024). Wetterhahn Science Symposium Posters 2024. 13.
https://digitalcommons.dartmouth.edu/wetterhahn_2024/13
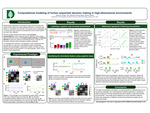
Restricted
Available to Dartmouth community via local IP address.